Abstract
- autoregressive transformer language model(LM)에서 factual associations(사실 관계)를 저장하는 장소와 이를 기억해내는 능력을 분석
causal intervention(= causal tracing):
- LM 내 어떤 module(= neural activations, layers, NN)이 model이 사실관계를 예측할때 결정적인 역할을 하는가?를 분석하기 위한 실험
- 결과를 통해 model의 중간 layer 내에 있는 feed-forward layers(MLP)가 subject tokens를 처리 할 때 하는 연산(computations)이 target(=object)를 prediction 할 때 영향을 끼치는 것을 밝혀 냄
- 이 computations가 정말 사실관계를 기억해내는 능력과 관련이 있는지 확인하기 위해 RANK-ONE-MODEL-EDITING(ROME)을 통해 feed-forward weights를 수정해서 저장된 사실관계를 update하는 실험을 추가로 진행
ROME (RANK-ONE-MODEL-EDITING)
- ROME은 standard zero-shot relation extraction (zsRE)에 효과적
- ROME을 specificity와 generalisation 모두 유지해야해서 모델 입장에서 다소 어려운 counterfacutal을 담고 있는 dataset을 가지고 evaluation을 추가로 진행
- 결과를 통해 mid-layer feed-forward modules가 사실관계를 저장하는데 중요한 역할을 하는것을 증명하고 computational mechanisms의 직접적인 수정을 할 수 있는 method를 제안 (model editing을 위한 실현가능한 approach가 될 수 있음)
1. Introduction
- 이 논문에서는 'large LM이 사실관계를 어디에 저장하는가?'에 대한 물음에 대한 답으로, GPT 내 일어나는 localised computation이 사실관계에 대응되고 직접수정이 가능하다는 증거를 제시함
- LLM은 사실관계를 예측할 수 있는 능력을 갖추고 있음
- e.g. "The space needle is located in the city of ___ " 라는 빈칸 문장이 주어졌을때, "Seattle"을 예측 할 수 있음
- 이 논문에서는 GPT 같은 autoregressive transformer model에 어떻게 사실관계가 저장되는가에 대한 실험을 함
- BERT와 같은 masked models에 대한 연구는 어느정도 진행됐는데, unidirectional attention & generation capabilities에 대한 연구를 한다는 점에서 기존 연구와는 다름
experiment 1: causal tracing
- 하나의 subject와 관련된 사실정보를 떠올리는데 영향을 끼치는 특정한 modules를 찾기위해 원인 영향 분석(causal mediation analysis)을 통해 GPT 내부에 있는 hidden state activations의 영향도(causal effects)를 추적
- feed-forward MLP가 subject해당하는 tokens 중 마지막 token을 처리할 때 결정적인 역할을 한다는 것을 밝혀냄
experiment 2: ROME
- ROME을 도입하여 model weights에 FFN의 행동을 결정하는 parameters를 수정하여 위에서 발견한 점들을 test
- 평가를 통해 mid-layer MLP modules가 표면적인 관계(specific surface forms)를 넘어서 일반화할 수 있는 사실관계를 저장하고, subject에 specific한 상태를 유지하는 것을 확인
- ROME이 다른 model editing approaches와 비슷한 성능을 발휘한다는 것을 szRE를 통해 확인할 수 있었음
- 더 어려운 case에 대한 ROME의 영향을 평가하기 위해, counterfactual assertions가 담긴 새로운 dataset 도입
2. Interventions on Activations for Tracing Information Flow
- 각각의 사실을 예측하는데 가장 큰 causal effect를 미치는 특정 hidden states를 분석하고 식별하기 위해, 첫번째로 facts가 저장된 위치정보를 찾음
data
- fact = knowledge tuple로 표현 (subject s, relation r, object o)
model
- qutoregressive transformer language model (GPT) G에 s와 r을 유도하기 위해, s와 r을 표현하는 prompt p를 도입하여 o를 맞게 예측하는지 실험
- G:X→Y
- V: vocabulary
- x: token sequences
- y: probability distribution
- prediction: next-token continuations of x
internal computation
h(l)i=h(l−1)i+a(l)i+m(l)i
a(l)i=attn(l)(h(l−1)1,h(l−1)2,…,h(l−1)i)
m(l)i=W(l)projσ(W(l)fcγ(a(l)i+h(l−1)i))
- h(l)i: a series of hidden state vector (witin transformer layer l and at ith token)
- a(l)i: global attention
- m(l)i: local MLP
2.1. Causal Tracing of Facutal Associations
- 올바른 사실을 예측하는데 각각의 states가 기여하는 바를 계산하기 위해, 모델 G를 세가지 방법으로 run 시켜서 내부의 activations를 수집 및 분석함
- 1) 올바른 fact를 예측하는 clean run
- 2) 결과값이 손상된 corrupted run
- 3) 하나의 stat가 가진 prediction을 복원하는 능력을 test하기 위한 corrupted-with-restoration
clean run:
- factual prompt x를 G에 통과시키고 모든 hidden activations {h(l)i|i∈[1,T],l∈[1,L]}을 수집
- e.g. prompt: "The Space Needle is in downtown _____", o: "Seattle"
corrupted run:
- G를 run 시키기 전에 subject에 해당하는 token에 noise를 추가
- x는 embedding layer를 통과하여 [h(0)1,h(0)2,…,h(0)T]로 embedded됨
- h(0)i:=h(0)i+ϵ: 여기에 noise ϵ를 추가하여 input 값을 임의로 바꿈
- 그 다음에 corrupted activations {h(l)i∗|i∈[1,T],l∈[1,L]}를 수집
- subject에 추가된 noise 때문에 정답을 예측할 확률은 낮아짐
corrupted-with-restoration run:
- corrupted run처럼 모델을 실행시키다가 token ˆi and layer l에서 clean activation인 h(l)ˆi을 출력하도록 강제함
- 나머지 계산은 원래대로 진행
- 몇몇의 clean states는 결과를 correct fact로 이끌 것이고, 이는 causal importance를 나타낼것
metric
- P[0], P∗[0], P∗,cleanh(l)i[0]: clean, corrupted, and corrupted-with-restoration run 이후 o를 예측할 확률
- total effect: TE=P[0]−P∗[0]
- indirect effect: IE=P∗,cleanh(l)i[0]−P∗[0]
- average total effect: ATE
- average indirect effect: AIE
2.2. Causal Tracing Results
- 문장 내 위치와 components(individual states, MLP layers, attention layers)를 이용해서 조건을 다양하게 설정하여 ATE 18.6% 산출 (Figure 2)
- prediction 직전 위치 (late site)에 causal states가 높게 등정된건 어떻게 보면 당연한 일인데, 그에 반해 15th layer 내 subject의 마지막 token(early site)에서 AIE값이 높게 나왔다는건 새로운 발견임
- causal effects에 대한 기여도를 MLP와 attention 모듈 관점에서 나눴을 때, MLP가 early site에서 결정적인 역할을 한다는 것을 보여줌
figure 3
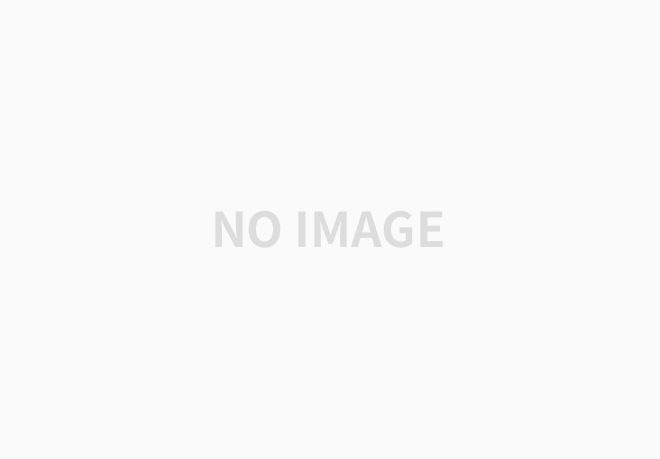
early site에서 MLP module이 하는 역할을 알아보기 위해 modified causal graph로 간접적인 영향도를 분석함
(a) 각 모듈의 contribution을 corrupted input을 이용해서 측정
(b) causal effects를 측정할 때 MLP modules의 효과를 분리시키기 위해 ith token에서 MLP modules를 잘라내고 corrupted state 상태로 freezing 시킴으로써, clean state의 추가에 영향을 받지 않게됨
(c) MLP가 절단된 모델과 original 모델의 AIE 비교
(d) MLP를 잘라낸 모델의 lowest layers는 causal effects를 잃게됨
(f) 그에 반해 highest layers의 causal effects는 MLP의 존재여부에 큰 영향을 받지 않음
(e) 이 결과는 MLP가 middle layers에서 fact 정보를 기억해내는데 중요한 역할을 함을 알 수 있음
가설: "localised midlayer MLP가 key-value mapping 역할을 하면서 subject에 대한 사실정보를 떠올린다"
2.3. The Licalised Factual Association Hypothesis
- 3.2.에서의 causal traces의 결과로 사실관계정보를 저장하기 위한 특정한 machanism을 사실이라고 상정함
- 각각의 midlayer MLP modules는 subject를 이노딩한 input을 받고, 해당 subject에 관해 기억하고 있는 속성을 출력
- middle layer MLP outputs은 정보를 축적하고, 더해진 정보는 high layers에 있는 attention에 의해 마지막 token으로 복사됨
- 이 가설은 사실관계가 저당된 위치를 3개의 dimension에 걸쳐 국한시킴: 1) MLP modules 2) 특정한 middle layers 3) subject의 마지막 token을 처리할때 (다른 연구들과도 일치)
- 가설을 test하기 위해 중간 레이어 l∗에 있는 하나의 MLP module을 집중적으로 분석하여 임의의 fact 정보를 저장하기 위해 weights를 명시적으로 수정해도 되는지를 알아봄
'Data Science > Paper Review' 카테고리의 다른 글
CONTROL PREFIXES for Parameter-Efficient Text Generation (2021) 논문리뷰 (0) | 2023.05.13 |
---|---|
[P-tuning] GPT Understands, Too (2021) 논문리뷰 (2) | 2023.05.13 |
Prefix-Tuning: Optimizing Continuous Prompts for Generation (2021) 논문 리뷰 (1) | 2023.05.07 |
AdapterHub: A Framework for Adapting Transformers (2020) (0) | 2023.05.03 |
K-ADAPTER: Infusing Knowledge into Pre-Trained Models with Adapters (2020) (1) | 2023.05.02 |